Posted on: Thursday April 24, 2025
One prevailing comment I hear about my job, whether in person or via social media, is “your job sounds so cool!” And you know what? It is. But I’m about to reveal the dark underbelly of my job, the bane of my existence, the thing that frustrates me to my core: dirty data.
Last year, the Museum implemented a new Collections Management System (CMS) after years with an old database that was vintage in all the wrong ways. The worst part of our old database was the inability to control the data being entered into the system, resulting in dirty data in desperate need of attention.
What is dirty data?
Dirty data is incorrect data, misspellings, outdated or inaccurate information found in various fields in our database.
How does it happen?
One cause of dirty data is information changing over time, like the reclamation of First Nations place names or changes to the nomenclature we use to categorize objects, leading to inaccuracies in our existing data. However, the biggest culprit of dirty data in our system is human error. With hundreds of individuals creating or entering data into dozens of fields over a fifty-year period, mistakes are bound to happen. This ranges from simple spelling errors to lazy cataloguing resulting in variations in what should be one accurate piece of data.
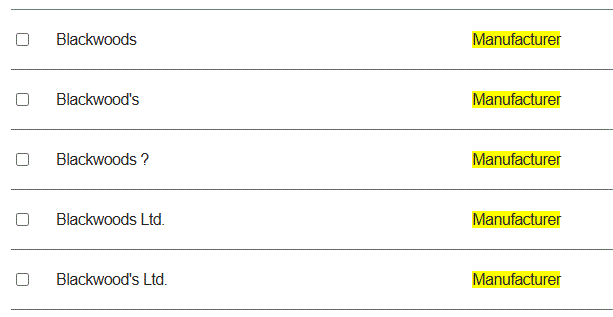
Here we see many variations on the name of a now-defunct Winnipeg-based beverage company: Blackwoods, Blackwood’s, Blackwoods Ltd., Blackwood’s Ltd. Over the years, different cataloguers have entered the manufacturer name in various ways, either due to information available to them, like the name ‘Blackwoods’ embossed on a bottle, or an error in judgement.
In case you’re dying to know, the correct name is Blackwoods Ltd.
Why is dirty data bad?
Dirty data is bad for a handful of reasons. One, the most detrimental to the function of the CMS, is reduced data quality – these errors mean that impacted objects are overlooked in searches of the system.
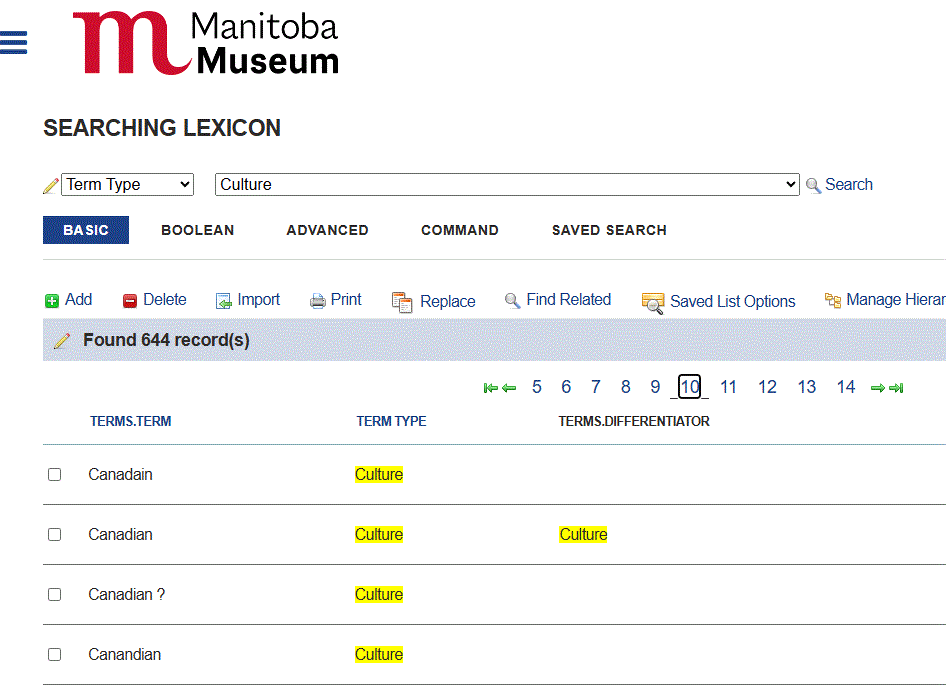
For instance, if I searched for Culture = Canadian, any records with the erroneous Canadain or Canandian in the Culture field would be missing from my results. Due to a typing error, we could be missing out on key objects that would be super relevant to a curator or researcher.
Another downside of dirty data is damage to our reputation. Errors in our collections data have an impact on how others may perceive us – if this particular data is incorrect, what else is incorrect? We have an obligation, as a museum, to maintain and provide accurate, up-to-date information about our collections.
How are you cleaning up your data?
We are currently in this phase of our new CMS implementation. I’m exporting data, reviewing it, making necessary changes, and then importing the cleansed data back into the database.
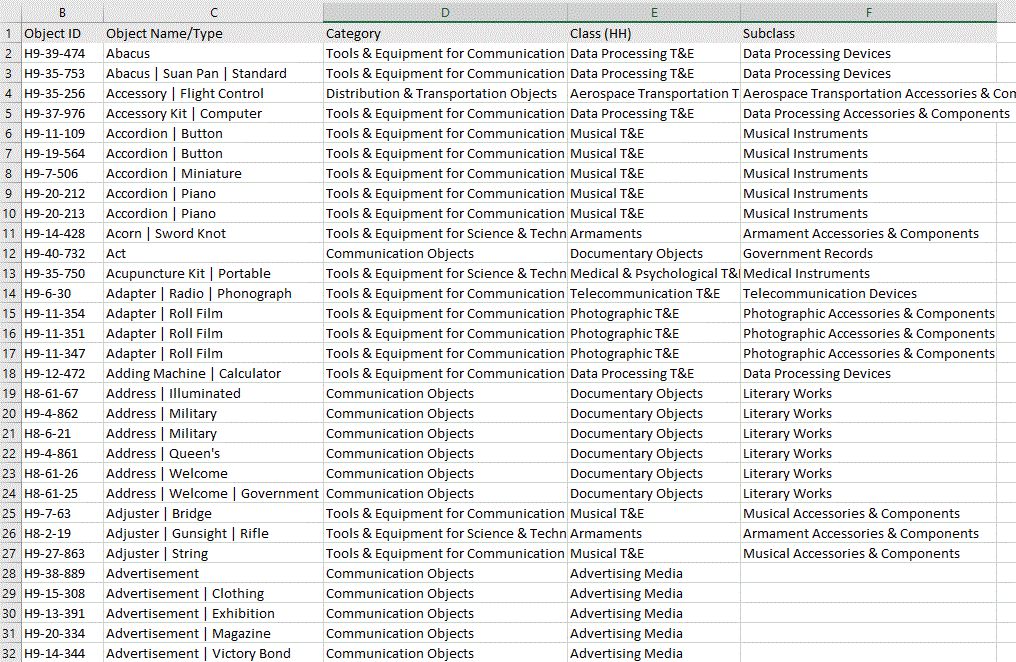
Here, I’m standardizing the nomenclature for our History collection. Our former database had Category and Class fields and now I’m also adding the tertiary Subclass dataset, which will allow us to get even more detailed in searches without having to exclusively use Object Name. For example, I can now search Drinking Vessels and get all the cups, mugs, tankards, glasses, teacups, tumblers, etc. in the collection instead of having to search individually for all these kinds of objects.
We also have a handy-dandy “search and replace” feature that the database “super users” (where’s my cape?!) can use to easily swap out one dataset for another.
What are we doing to prevent the creation of dirty data moving forward?
Our new collections management system has some key features that helps us prevent the entry of dirty data:
- We now have many lexicon-controlled fields, meaning that you need to pull data from a pre-approved list of available terms. No more Object Name=Tunbler because you’ll only be able to enter Object Name=Tumbler, for instance. We can update the lexicon terms available for those fields as needed, which is helpful.
- Our long text fields, like description or provenance, now have spellcheck! This is great for cataloguers who struggle with spelling. We’re living in 2025, folks!
Further to this, additional training and support for the creators of data is also a top priority moving forward. Will some dirty data still sneak in now and then? Absolutely. But then I’ll be there to scrub it clean.
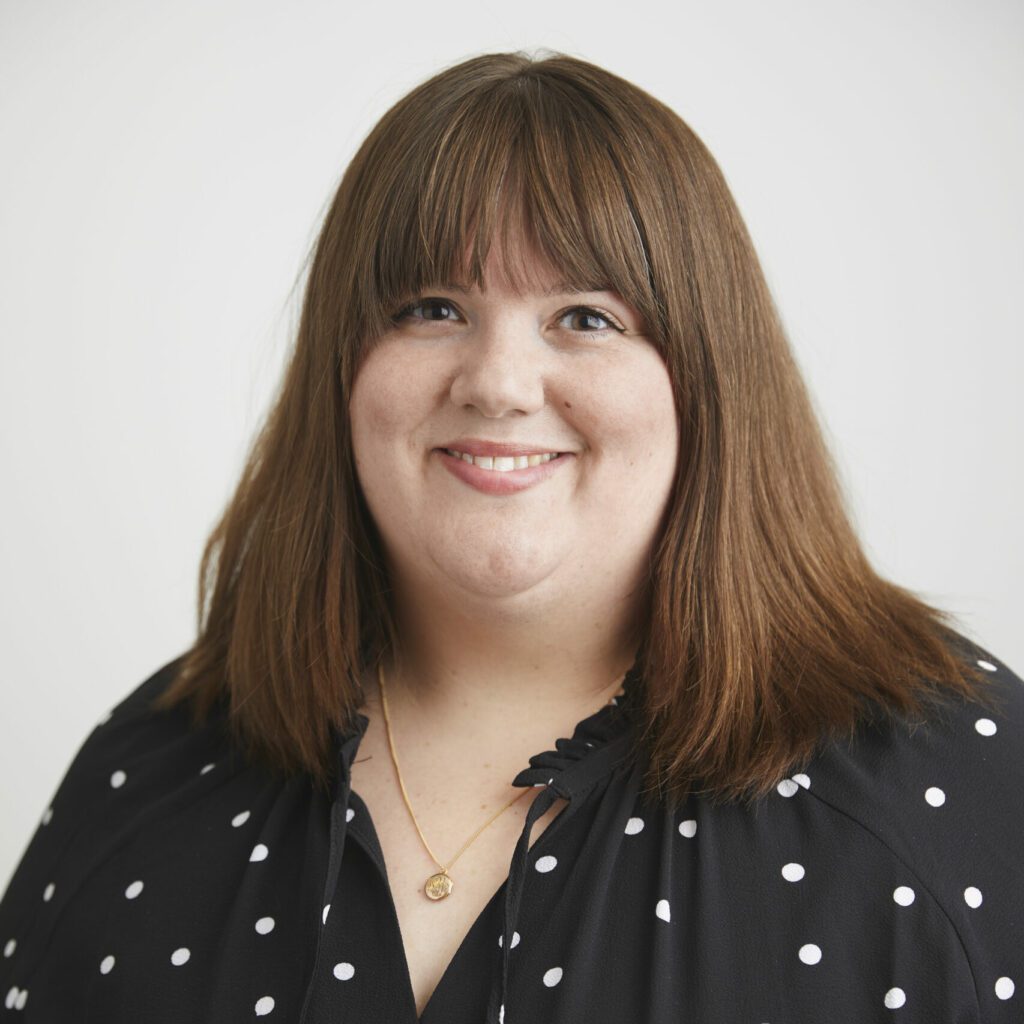